Reproducibility in Science
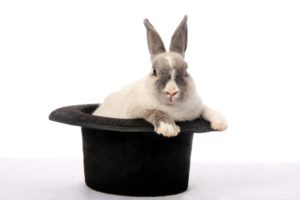
In 2011 a University of Virginia psychologist named Brian Nosek began the Reproducibility Project. He simply wanted to see if the reported problem with reproducing the scientific findings of published research studies in psychology was true. Nosek and his team recruited 250 research psychologists, many of whom volunteered their time to double-check what they considered to be the important works in their field. They identified 100 studies published in 2008 and rigorously repeated the experiments while in close consultation with the original authors. There was no evidence of fraud or falsification, but “the evidence for most published findings was not nearly as strong as originally claimed.”
Their results were published in the journal Science: “Estimating the Reproducibility of Psychological Science.” In a New York Times article about the study, Brian Nosek said: “We see this is a call to action, both to the research community to do more replication, and to funders and journals to address the dysfunctional incentives.” The authors of the journal article said they conducted the project because they care deeply about the health of psychology and believe it has the potential to accumulate knowledge about human behavior that can advance the quality of human life. And the reproducibility of studies that further that goal is central to that aim. “Accumulating evidence is the scientific community’s method of self-correction and is the best available option for achieving that ultimate goal: truth.”
The present results suggest that there is room to improve reproducibility in psychology. Any temptation to interpret these results as a defeat for psychology, or science more generally, must contend with the fact that this project demonstrates science behaving as it should. Hypotheses abound that the present culture in science may be negatively affecting the reproducibility of findings. An ideological response would discount the arguments, discredit the sources, and proceed merrily along. The scientific process is not ideological. Science does not always provide comfort for what we wish to be; it confronts us with what is.
The editor in chief of Science said: ““I caution that this study should not be regarded as the last word on reproducibility but rather a beginning.” Reproducibility and replication of scientific studies has been a growing concern. John Ioannidis of Stanford has been particularly vocal on this issue. His best-known paper on the subject, “Why Most Published Research Findings Are False,” was published in 2005. A copy of one of his latest works, “Empirical assessment of published effect sizes and power in the recent cognitive neuroscience and psychology literature,” can be found here. Szucs and Ioannidis concluded that false report probability was likely to exceed 50% for the whole literature. “In light of our findings the recently reported low replication success in psychology is realistic and worse performance may be expected for cognitive neuroscience. “
A recent survey conducted by the journal Nature found that more than 70% of researchers have tried and failed to reproduce another scientist’s experiments. More than half failed to reproduce their own experiments. In response to the question, “Is there a reproducibility crisis?” 52% said there was a significant crisis; another 38% said there was a slight crisis. More than 60% of respondents thought that two factors always or often contributed to problems with reproducibility—pressure to publish and selective reporting. More than half also pointed to poor oversight, low statistical power and insufficient replication in the lab. See the Nature article for additional factors.
There were several suggestions for improving reproducibility in science. The three most likely were: a better understanding of statistics, better mentoring/supervision, and a more robust experimental design. Almost 90% thought these three factors would improve reproducibility. But even the lowest-ranked item had a 69% endorsement. See the Nature article for additional approaches for improving reproducibility.
In “What does research reproducibility mean? John Ioannidis and his coauthors pointed out how one of the problems with examining and enhancing the reliability of research is that its basic terms—reproducibility, replicability, reliability, robustness and generalizability—aren’t standardized. Rather than suggesting new technical meanings for these nearly identical terms, they suggested using the term reproducibility with qualifying descriptions for the underlying construct. The three terms they suggested were: methods reproducibility, results reproducibility, and inferential reproducibility.
Methods reproducibility is meant to capture the original meaning of reproducibility, that is, the ability to implement, as exactly as possible, the experimental and computational procedures, with the same data and tools, to obtain the same results. Results reproducibility refers to what was previously described as “replication,” that is, the production of corroborating results in a new study, having followed the same experimental methods. Inferential reproducibility, not often recognized as a separate concept, is the making of knowledge claims of similar strength from a study replication or reanalysis. This is not identical to results reproducibility, because not all investigators will draw the same conclusions from the same results, or they might make different analytical choices that lead to different inferences from the same data.
They said what was clear is that none of these types of reproducibility can be assessed without a complete reporting of all relevant aspects of scientific design.
Such transparency will allow scientists to evaluate the weight of evidence provided by any given study more quickly and reliably and design a higher proportion of future studies to address actual knowledge gaps or to effectively strengthen cumulative evidence, rather than explore blind alleys suggested by research inadequately conducted or reported.
In “Estimating the Reproducibility of Psychological Science,” Nosek and his coauthors said it is too easy to conclude that successful replication means the original theoretical understanding is correct. “Direct replication mainly provides evidence for the reliability of a result.” Alternative explanations of the original finding may also account for the replication. Understanding come from multiple, diverse investigations giving converging support for a certain explanation, while ruling out others.
It is also too easy to conclude a failure to replicate means the original evidence was a false positive. “Replications can fail if the replication methodology differs from the original in ways that interfere with observing the data.” Unanticipated factors in the sample, setting, or procedure could alter the observed effect. So we return to need for multiple, diverse investigations.
Nosek et al. concluded that their results suggested there was room for improvement with reproducibility in psychology. Yet the Reproducibility Project demonstrates “science behaving as it should.” It doesn’t always confirm what we wish it to be; “it confronts us with what is.”
For more on reproducibility in science, also look at: “The Reproducibility Problem” and “’Political’ Science?” on this website.